Can Machine Learning Improve Cancer Care? Predictive Tools for Risk of Short-Term Mortality
December 9, 2019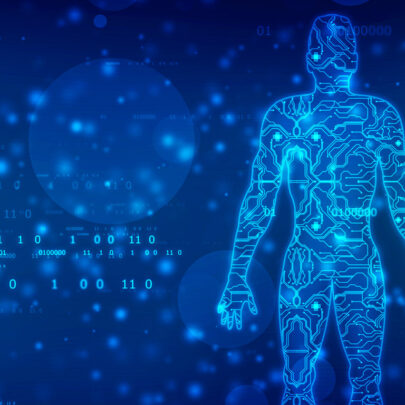
“How long do I have?”
It is the first question many patients ask after a cancer diagnosis. It is also among the hardest to answer. For decades, predicting cancer survival was more art than science. But now, unprecedented computing power and access to digital health information offer a tantalizing opportunity: can machine learning (ML) algorithms succeed where others fail?
A new study from LDI Fellows Ravi Parikh, Christopher Manz, Amol Navathe, Mitesh Patel and colleagues tackles the question head on. The authors applied several ML algorithms to electronic health records of more than 26,000 patients seen in outpatient oncology clinics in the University of Pennsylvania health system. In this group, 4% of patients died within six months. Among patients flagged as high risk by the most effective ML tool, 51.3% died within six months, compared to 3.1% for those flagged as low-risk.
Machine learning in real time
Does this statistical validity translate into clinical usefulness? To begin to explore this question, the researchers deployed a ML tool in real time for four weeks and surveyed 15 physicians who assessed 171 patients flagged by the algorithm as appropriate for a serious illness conversation. The physicians agreed with the algorithm 58.8% of the time—in their assessment, 100 of the 171 patients were high risk and good candidates for advanced care planning. The level of agreement between physicians and ML algorithms suggests that these ML tools may be a useful first step for identifying candidates for these crucial conversations.
How should we interpret these results? First, artificial intelligence cannot divine the future, and it likely never will. While ML tools can accurately identify patients at higher risk of short-term adverse outcomes, they are not fortune tellers. About half of patients classified as high risk died within six months, compared to less than 1 in 25 in the low-risk cohort. While this is an improvement over most existing statistical methods, it does not provide a succinct answer to the question, “How long do I have?”
First screener status
Second, while ML algorithms may be the first screener for which patients might benefit the most from earlier advanced care planning conversations, physicians likely have access to less tangible factors that make those consultations more or less appropriate, such as information about family preferences. For the foreseeable future, there will be no robot doctors, but machine learning can be integrated as a decision support tool for physicians and patients.
The case for using ML prediction tools is clear. Currently, most patients with cancer who die do not have end-of-life planning consultations. Without advanced care planning, care often diverges from patients’ goals. With more accurate identification of high-risk patients, care can become more patient-centered.
Deploying predictive analytics
Previously, Navathe and Parikh wrote about how to effectively regulate and deploy machine learning and predictive analytics in medicine. Some of the proposals put forward before resonate here. Predictive analytics should be tied to real clinical decisions and interventions. In this case, ML algorithms predict risk of an outcome of importance to patients—death—and can be useful for guiding clinical decisions (whether to begin end-of-life counseling). While an algorithm will not perfectly predict survival from cancer, they can serve as tools to streamline the identification of high-risk patients, adding value to the very human interaction that lies at the heart of clinical care.
Co-posted from Penn LDI