Recognizing and Addressing Bias in Health Care AI/Algorithms
November 25, 2019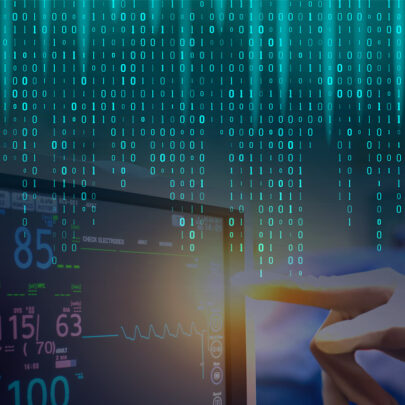
In a Viewpoint article in the Journal of the American Medical Association (JAMA), three University of Pennsylvania researchers affiliated with the Penn Center for Cancer Care Innovation (PC3I) discuss potential ways to counter or correct clinical algorithm bias.
Ravi Parikh, MD, MPP, Amol Navathe, MD, PhD, and Stephanie Teeple, an MD-PhD Candidate, write in their conclusion:
Because of its reliance on historical data, which are based on biased data generation or clinical practices, Artificial Intelligence can create or perpetuate biases that may worsen patient outcomes. However, by strategically deploying AI and carefully selecting underlying data, algorithm developers can mitigate AI bias.
Passing biases on
In the body of their JAMA paper entitled “Addressing Bias in artificial Intelligence in Health Care,” the trio details a number of methods that might be used to counter algorithm bias in clinical AI settings. One widely-recognized problem is that large swaths of medical literature and various record collections that are used as the “big data” that algorithms feed on, contain racial, gender and other kinds of biases that can be passed on.
As the use of AI continues to spread throughout the diagnostic, decision support and therapeutic areas of the health care, the issue of how such “baked in” biases may impact the decision-making software systems’ conclusions has become a serious concern for clinicians, hospital administrators and insurance companies alike.
Importance of unbiased source data
Major national headlines have recently drawn attention to study findings showing how racial bias skews algorithmic systems already in wide clinical use, potentially affecting the care of millions of patients. However, racial “bias” is only one of many ways AI can get it wrong if not provided correct source data. The authors emphasize that a clear solution to this problem is to pay greater attention to using unbiased source data.
They also point out that the power of AI itself could be used to do things like identify real-time bias in physician decision making, such as “flagging a questionable opioid prescription at the end of a primary care clinician’s day, providing a needed check on this decision.”