Nudges Plus Machine Learning Triple Advanced Care Conversations
October 16, 2020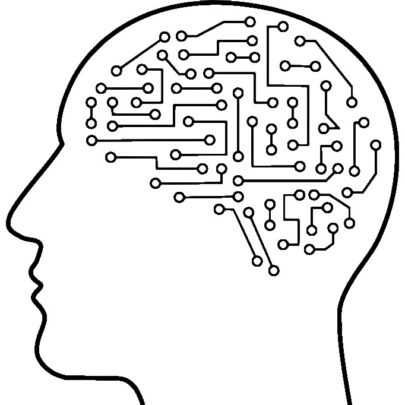
A new prospective, randomized study of nearly 15,000 patients with cancer published in JAMA Oncology found an electronic nudge to clinicians—triggered by an algorithm that used machine learning methods to flag patients who would most benefit from a conversation around end-of-life goals—significantly increased the rate of those discussions. The intervention led to a quadrupling of serious illness conversations for all patient encounters and a tripling for patients having high predicted mortality risk.
Early and frequent conversations with patients suffering from serious illnesses, particularly cancer, have been shown to increase satisfaction, quality of life, and care that’s consistent with their values and goals. However, today many do not get the opportunity to have those discussions with a physician or loved ones before their disease has progressed too far and they’re too ill.
“Within and outside of cancer, this is one of the first real-time applications of a machine learning algorithm paired with a prompt to actually help influence clinicians to initiate these discussions in a timely manner, before something unfortunate may happen,” said co-lead author Ravi B. Parikh, MD, an Innovation Faculty member at the Penn Center for Cancer Care Innovation (PC3I). “And it’s not just high-risk patients. It nearly doubled the number of conversations for patients who weren’t flagged—which tells us it’s eliciting a positive cultural change across the clinics to have more of these talks.”
In a separate JAMA Oncology published in September, the research team validated the Penn Medicine-developed machine learning tool’s effectiveness at predicting short-term mortality in patients in real-time using clinical data from the electronic health record (EHR). The algorithm considers more than 500 variables—age, hospitalizations, and co-morbidities, for example—from patient records, all the way up until their appointment. That’s one of the advantages of using the EHR to identify patients who may benefit from a timely conversation. It’s in real time, as opposed to using claims or other types of historical data to make predictions.
“We’ve taken an algorithm from retrospective validation to real-time validation to actually testing it in the clinic to see if it can shape patient care.”
“Because of its success,” Parikh shared, “I think we’ve provided of a road map for other institutions that may be thinking of using analytics to drive important behaviors.”
Christopher Manz, MD, of the Dana Farber Cancer Institute, who was an Innovation Fellow at PC3I at the time of the study, serves as co-lead author. Other PC3I co-authors include PC3I Director Justin Bekelman, MD; Governing Board member Lynn Schuchter, MD; Advisory Board members Larry Shulman, MD, and Mitesh Patel, MD, MBA, MS; and Affiliated faculty Dylan Small, PhD.
The authors go into more detail in an opinion article in STAT News. You can read more at the Penn Medicine news release.
Manz CR, Parikh RB, Small DS, et al. Effect of Integrating Machine Learning Mortality Estimates With Behavioral Nudges to Clinicians on Serious Illness Conversations Among Patients With Cancer: A Stepped-Wedge Cluster Randomized Clinical Trial. JAMA Oncol. Published online October 15, 2020. doi:10.1001/jamaoncol.2020.4759